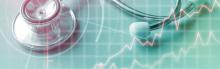
Salvatory R. Kessy, Michael Sherris, Andrés M. Villegas and Jonathan Ziveyi
Abstract: We present a stacked regression ensemble method that optimally combines dierent mortality models to reduce the mean squared errors of mortality rate forecasts and mitigate model selection risk. Stacked regression uses a supervised machine learning algorithm to approximate the horizon-specific weights by minimizing the cross-validation criterion for each forecasting horizon. The horizon-specific weights facilitate the development of a mortality model combination customized to each horizon. Unlike other model combination methods, stacked regression simultaneously solves model selection and estimates model combinations to improve model forecasts. Our numerical illustrations based on 44 populations from the Human Mortality Database demonstrate that stacking mortality models increases predictive accuracy. Using one-year-ahead to 15-year-ahead out-of-sample mean squared errors, we find that stacked regression improves mortality forecast accuracy by 13% - 49% and 19% - 90% over the individual mortality models for males and females, respectively. Therefore, combining the mortality rate forecasts provides lower out-of-sample point forecast errors than selecting the single best individual mortality method. Stacked regression ensemble also achieves better predictive accuracy than other model combination methods, namely Simple Model Averaging, Bayesian Model Averaging, and Model Confidence Set. Our results support the stacked regression ensemble approach over individual mortality models and other model combination methods in forecasting mortality rates. We also provide a user-friendly open-source R package, CoMoMo, that combines multiple mortality rate forecasts using dierent model combination techniques.
Keywords: Stacked regression, ensemble learning, cross-validation, model uncertainty, model combination, age-period-cohort model, mortality forecasting.
